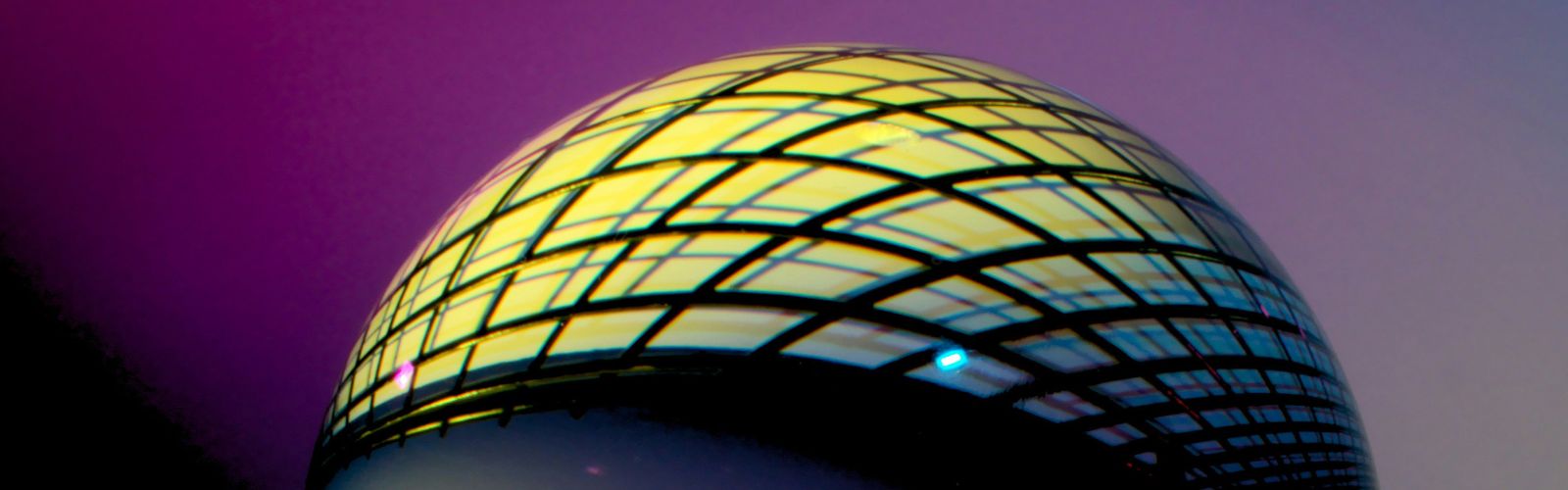
V7 Labs – MLOps For Vision AI
What do Merck, GE Healthcare, Fujifilm, Continental, Tractable and hundreds of customers have in common? They run their vision AI on London-based V7 Labs, the latest addition to our portfolio.
There are over $100 billion worth of tasks performed by visual decision making and AI has the potential to deliver additional global economic activity of around $13 trillion by 2030.
Businesses are adopting AI technology to automate decision-making and benefit from new business opportunities, but it is far from easy. Most have little to no idea where to put the unstructured data they collect, let alone label it and turn it into services. Data annotation and model training are the most challenging limitations to AI adoption at scale – and both are solved by V7.
V7 empowers AI teams with automated training data workflows. The platform accelerates the creation of high-quality training data by up to 100x. It accomplishes this by giving users the ability to build automated image and video data pipelines, organise complex datasets and train and deploy state-of-the-art vision AI models while managing their lifecycle in production.
V7 Labs joins the Amadeus portfolio of human-computer interfaces – from vision (Tobii, Quibim, Five, Slamcore, XYZ Reality, Pimloc, Natrox) to voice (PolyAI, VocalIQ, Entropic, XMOS, Speechmatics) – along with MLOps and AI-infrastructure specialists (Seldon, Improbable, Graphcore, Brytlyt).
Data-first approach to building robust AI systems
AI requires companies to continuously collect, organise and label image data so that AI models may adapt to new scenarios, camera models or objects. Previously, AI companies would build these systems in-house, having to maintain them alongside rapid developments in machine learning. They are now switching to V7, which has condensed, into a single SaaS platform, best practices for organising datasets, labelling them autonomously and monitoring the status of AI models.By building on V7, companies can iterate faster and have confidence in the robustness of their production processes.
Models learn from datasets. If they are close to where these reside, they can continually learn from new data, and point out any training images that have skewed their performance over time, allowing for teams working on accuracy-critical models to learn when and why an AI will fail. V7 allows teams to train and run deep learning models in the cloud on managed GPU servers, removing the need for dev-ops work.
V7 enables monitoring of this data’s life cycle, as it gets captured, automatically labelled using AI or humans and sent off to further improve an AI’s learning. If the proliferation of ML has taught us anything, it is that turning your AI data’s lifecycle into a flexible end-to-end workflow is key. V7 is creating the finest platform for training data out there, with built-in AI models that outclass the previous state-of-the-art.
Demand is being intensified by the growing number of deep learning models in production and the need for automation. Healthcare data is the fastest area of growth, whilst the largest by volume is expert visual inspection, ensuring that power lines, oil pipelines and other critical infrastructure does not have rust, leaks or damage.
There is much more to come for V7 users in 2021 to bridge the gap between training data and AI model performance.
Every click counts
V7’s platform offers a uniquely powerful capability called Auto-Annotate, which can segment any object to turn it into an AI-learnable annotation. Auto-Annotate is trained on over 10 million images of varying nature, determined by V7 to be of high accuracy. This way it can work on anything ranging from medical images like x-rays, to satellite imagery and damaged cars.
Auto-Annotate is the most accurate AI for automated segmentation of any arbitrary object, recently benchmarked on popular computer vision datasets and performing 96.7% IoU score on Grabcut using 2 clicks, and 90.6% on the DAVIS challenge with 3.1 clicks. It outperforms (in IoU and speed) high-budget R&D projects like Nvidia’s PolyRNN++ and Curve GCN. Unlike its academic competitors, it’s class agnostic, meaning that it can segment any object without training, and it is continuously learning from user input. It can segment the bones of a hammerhead shark just as well as it segments a taxi.
The ethos is automation first. Auto-Annotate, which runs in the cloud 24/7, gets you 10,000 segmentation labels in 5 hours, meaning you can build a proof of concept training set in a single day. It’s being used to produce the equivalent of 500 human labellers on a daily basis. V7 Darwin allows for a real-time learning of features, meaning that it starts learning from the very first image. Rather than building an API for human annotation, or a canvas for adding bounding boxes, they focused on accelerating throughput with active learning. This means starting with a small dataset, training, automating, and doing 20% less work on the next cycle. Repeat, and the gains compound.
The platform enables 12 types of annotation and unique scientific data formats, like DICOM medical imaging, SVS (used in digital pathology, which can reach sizes of 100,000 pixels squared) and 4K video. Machine learning teams can add hundreds of polygon annotations to represent objects in their training data on V7, which can hold over 2 million data points in a single image without lag on a common web browser.
V7 gives ML teams the chance to search through million-image datasets for image data to visualize their labels. Datasets can be versioned to exclude potentially biasing samples, and every label added is logged to keep an accurate record of the evolution of training data. The dataset management also enables customizable flows through which each image will go, as humans or AI models apply labels, before an image can be considered complete and ready for training. All datasets start with a largely human-driven annotation flow, and automate introducing AIs as the training data expands.
And now for the big question… the name
The founders will tell you that V7 was named after the areas of the visual cortex. “During the Cambrian explosion 500 million years ago, life developed sight, which it continued to refine through evolution, accelerating its diversity of skills. The human brain has six distinguishable areas: V1 through V6. We want to create a hypothetical 7th area meant for human-computer interfaces, and bring forth a similar burst of evolutionary capabilities to those of when life began to see”. No less.